Flink 官网主页地址:https://flink.apache.org
Flink 官方中文地址:https://nightlies.apache.org/flink/flink-docs-stable/zh/
1、状态管理
1.1、Flink 中的状态
1.1.1、概述
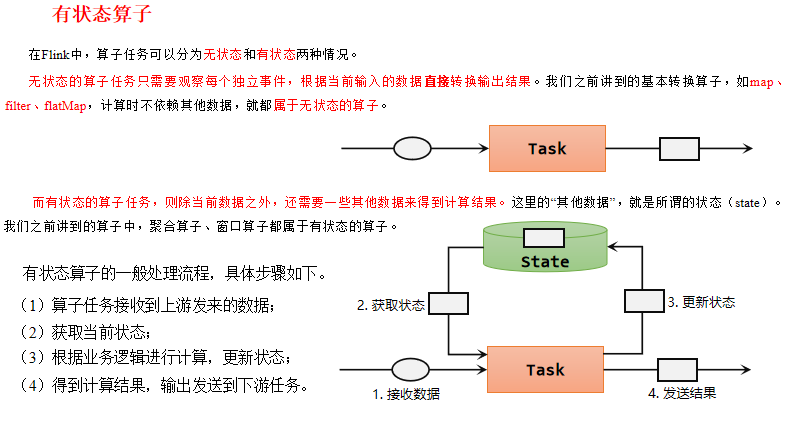
1.1.2、状态的分类
1、托管状态(Managed State)和原始状态(Raw State)
Flink 的状态有两种:托管状态(Managed State)和原始状态(Raw State)。
- 托管状态就是由 Flink 统一管理的,状态的存储访问、故障恢复和重组等一系列问题都由 Flink 实现,我们只要调接口就可以;
- 原始状态则是自定义的,相当于就是开辟了一块内存,需要我们自己管理,实现状态的序列化和故障恢复。
通常我们采用 Flink 托管状态来实现需求。
2、算子状态(Operator)和按键分区状态(Keyed State)
接下来我们的重点就是托管状态(Managed State)。
我们知道在 Flink 中,一个算子任务会按照并行度分为多个并行子任务执行,而不同的子任务会占据不同的任务槽(task slot)。由于不同的 slot 在计算资源上是物理隔离的,所以 Flink 能管理的状态在并行任务间是无法共享的,每个状态只能针对当前子任务的实例有效。
而很多有状态的操作(比如聚合、窗口)都是要先做 keyBy 进行按键分区的。按键分区之后,任务所进行的所有计算都应该只针对当前 key 有效,所以状态也应该按照 key 彼此隔离。在这种情况下,状态的访问方式又会有所不同。
基于这样的想法,我们又可以将托管状态分为两类:算子状态和按键分区状态。
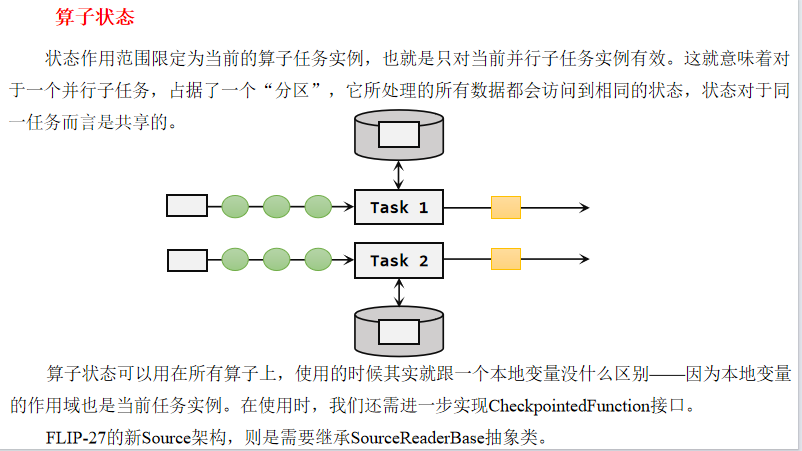
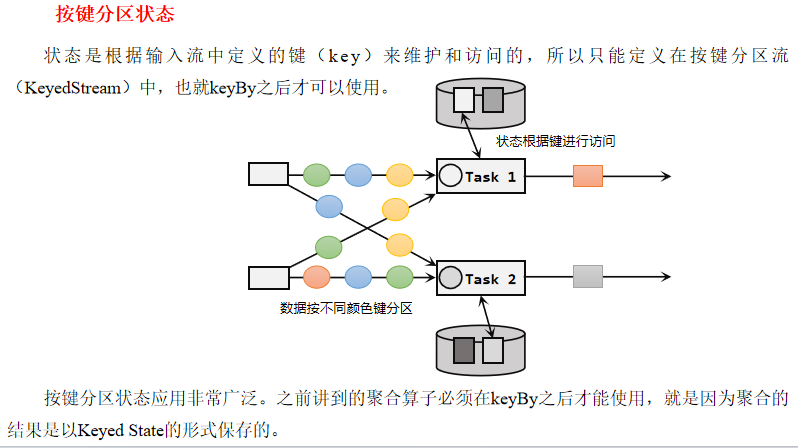
另外,也可以通过富函数类(Rich Function)来自定义 Keyed State,所以只要提供了富函数类接口的算子,也都可以使用 Keyed State。所以即使是 map、filter 这样无状态的基本转换算子,我们也可以通过富函数类给它们“追加”Keyed State。比如 RichMapFunction、RichFilterFunction。在富函数中,我们可以调用 .getRuntimeContext()
获取当前的运行时上下文(RuntimeContext),进而获取到访问状态的句柄;这种富函数中自定义的状态也是 Keyed State。从这个角度讲,Flink 中所有的算子都可以是有状态的。
无论是 Keyed State 还是 Operator State,它们都是在本地实例上维护的,也就是说每个并行子任务维护着对应的状态,算子的子任务之间状态不共享。
1.2、按键分区状态(Keyed State)
按键分区状态(Keyed State)顾名思义,是任务按照键(key)来访问和维护的状态。它的特点非常鲜明,就是以 key 为作用范围进行隔离。
需要注意,使用 Keyed State 必须基于 KeyedStream。没有进行 keyBy 分区的 DataStream,即使转换算子实现了对应的富函数类,也不能通过运行时上下文访问 Keyed Stat
1.2.1、值状态(ValueState)
顾名思义,状态中只保存一个“值”(value)。ValueState 本身是一个接口,源码中定义如下:
1 2 3 4
| public interface ValueState<T> extends State { T value() throws IOException; void update(T value) throws IOException; }
|
这里的 T 是泛型,表示状态的数据内容可以是任何具体的数据类型。如果想要保存一个长整型值作为状态,那么类型就是 ValueState。
我们可以在代码中读写值状态,实现对于状态的访问和更新。
- T value():获取当前状态的值;
- update(T value):对状态进行更新,传入的参数value就是要覆写的状态值。
在具体使用时,为了让运行时上下文清楚到底是哪个状态,我们还需要创建一个“状态描述器”(StateDescriptor)来提供状态的基本信息。例如源码中,ValueState 的状态描述器构造方法如下:
1 2 3
| public ValueStateDescriptor(String name, Class<T> typeClass) { super(name, typeClass, null); }
|
这里需要传入状态的名称和类型——这跟我们声明一个变量时做的事情完全一样。
案例
1 2 3 4 5 6 7 8 9 10 11 12 13 14 15 16 17 18 19 20 21 22 23 24 25 26 27 28 29 30 31 32 33 34 35 36 37 38 39 40 41 42 43 44 45 46 47 48 49 50 51
| ** * keyedState在使用时,只需要先keyBy * 在后续的处理函数中,自带生命周期方法 * open():需要再Task启动时,从之前的备份中根据描述取出状态 * * 特点:每一个 Task 上,各种 key 各有各的 State,互不干扰 * ------------------------------------------------ * ValueState 储存单个值,可以是任意类型 * ------------------------------------------------- * 检测每种传感器的水位值,如果连续的两个水位值超过 10 就输出报警 */ public class Demo01_ValueState { public static void main(String[] args) throws Exception { Configuration configuration = new Configuration(); configuration.setInteger("rest.port",3333); StreamExecutionEnvironment env = StreamExecutionEnvironment.getExecutionEnvironment(configuration);
env.socketTextStream("hadoop102",9999) .map(new WaterSensorFunction()) .keyBy(WaterSensor::getId) .process(new KeyedProcessFunction<String, WaterSensor, String>() {
private ValueState<Integer> state;
@Override public void open(Configuration parameters) throws Exception { ValueStateDescriptor<Integer> stateDescriptor = new ValueStateDescriptor<>("state", Integer.class); state = getRuntimeContext().getState(stateDescriptor); }
@Override public void processElement(WaterSensor value, KeyedProcessFunction<String, WaterSensor, String>.Context ctx, Collector<String> out) throws Exception { Integer lastVc = state.value();
if (lastVc!=null&&lastVc>10&&value.getVc()>10){ out.collect(ctx.getCurrentKey()+"连续两个传感器的vc("+lastVc+","+value.getVc()+")超过10....."); } state.update(value.getVc()); } }).print();
env.execute(); } }
|
测试截图:

1.2.2、列表状态(ListState)
将需要保存的数据,以列表(List)的形式组织起来。在 ListState 接口中同样有一个类型参数 T,表示列表中数据的类型。ListState 也提供了一系列的方法来操作状态,使用方式与一般的List非常相似。
- Iterable get():获取当前的列表状态,返回的是一个可迭代类型 Iterable;
- update(List values):传入一个列表 values,直接对状态进行覆盖;
- add(T value):在状态列表中添加一个元素 value;
- addAll(List values):向列表中添加多个元素,以列表 values 形式传入。
类似地,ListState 的状态描述器就叫作 ListStateDescriptor,用法跟 ValueStateDescriptor 完全一致。
1 2 3 4 5 6 7 8 9 10 11 12 13 14 15 16 17 18 19 20 21 22 23 24 25 26 27 28 29 30 31 32 33 34 35 36 37 38 39 40 41 42 43 44 45 46 47 48 49 50 51 52 53 54 55 56 57 58 59 60 61 62 63 64 65 66 67 68 69 70 71 72 73 74 75 76 77 78 79 80 81 82 83 84 85 86 87 88 89 90 91 92 93 94 95 96
|
public class Demo02_ListState { public static void main(String[] args) throws Exception { Configuration configuration = new Configuration(); configuration.setInteger("rest.port",3333); StreamExecutionEnvironment env = StreamExecutionEnvironment.getExecutionEnvironment(configuration);
env.socketTextStream("hadoop102",9999) .map(new WaterSensorFunction()) .keyBy(WaterSensor::getId) .process(new KeyedProcessFunction<String, WaterSensor, String>() {
private ListState<Integer> state;
@Override public void open(Configuration parameters) throws Exception { ListStateDescriptor<Integer> listStateDescriptor = new ListStateDescriptor<>("state", Integer.class); state = getRuntimeContext().getListState(listStateDescriptor); }
@Override public void processElement(WaterSensor value, KeyedProcessFunction<String, WaterSensor, String>.Context ctx, Collector<String> out) throws Exception {
state.add(value.getVc());
List<Integer> top3 = StreamSupport.stream(state.get().spliterator(), true).sorted(Comparator.reverseOrder()).limit(3).collect(Collectors.toList());
out.collect(ctx.getCurrentKey()+"最新Top水位:"+top3);
state.update(top3);
} }).print();
env.execute(); } public static class MyMapFunction implements MapFunction<String ,String>, CheckpointedFunction {
private ListState<String> strs; private ListState<String> strs1; private ListState<String> strs2; @Override public String map(String value) throws Exception { strs.add(value); return strs.get().toString(); }
@Override public void snapshotState(FunctionSnapshotContext context) throws Exception { System.out.println("MyMapFunction.snapshotState"); }
@Override public void initializeState(FunctionInitializationContext context) throws Exception { System.out.println("MyMapFunction.initializeState");
OperatorStateStore operatorStateStore = context.getOperatorStateStore(); ListStateDescriptor<String> strsListStateDescriptor = new ListStateDescriptor<>("list1", String.class); strs = operatorStateStore.getListState(strsListStateDescriptor);
} } }
|
测试截图:
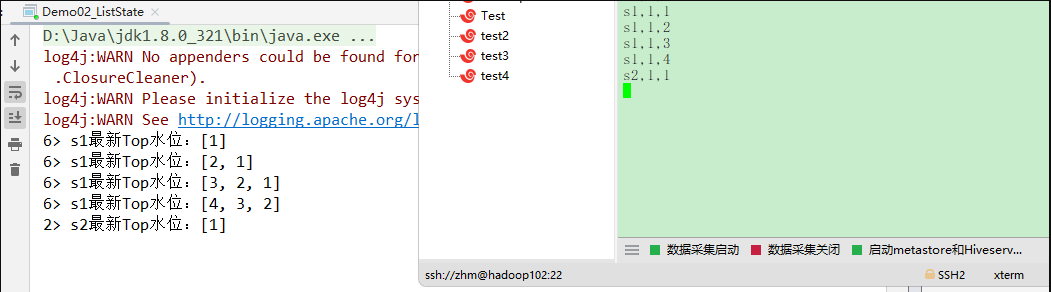
1.2.3、Map 状态(MapState)
把一些键值对(key-value)作为状态整体保存起来,可以认为就是一组 key-value 映射的列表。对应的 MapState<UK, UV>
接口中,就会有 UK、UV 两个泛型,分别表示保存的 key 和 value 的类型。同样,MapState 提供了操作映射状态的方法,与 Map 的使用非常类似。
- UV get(UK key):传入一个 key 作为参数,查询对应的 value 值;
- put(UK key, UV value):传入一个键值对,更新 key 对应的 value 值;
- putAll(Map<UK, UV> map):将传入的映射 map 中所有的键值对,全部添加到映射状态中;
- remove(UK key):将指定 key 对应的键值对删除;
- boolean contains(UK key):判断是否存在指定的 key,返回一个 boolean 值。另外,MapState 也提供了获取整个映射相关信息的方法;
- Iterable<Map.Entry<UK, UV>> entries():获取映射状态中所有的键值对;
- Iterable keys():获取映射状态中所有的键(key),返回一个可迭代 Iterable 类型;
- Iterable values():获取映射状态中所有的值(value),返回一个可迭代 Iterable 类型;
- boolean isEmpty():判断映射是否为空,返回一个 boolean 值。
案例
1 2 3 4 5 6 7 8 9 10 11 12 13 14 15 16 17 18 19 20 21 22 23 24 25 26 27 28 29 30 31 32 33 34 35 36 37 38 39 40 41 42 43 44 45 46 47 48 49 50 51
|
public class Demo03_MapState { public static void main(String[] args) throws Exception { Configuration configuration = new Configuration(); configuration.setInteger("rest.port",3333); StreamExecutionEnvironment env = StreamExecutionEnvironment.getExecutionEnvironment(configuration);
env.socketTextStream("hadoop102",9999) .map(new WaterSensorFunction()) .keyBy(WaterSensor::getId) .process(new KeyedProcessFunction<String, WaterSensor, String>() {
private MapState<Integer,Integer> state;
@Override public void open(Configuration parameters) throws Exception { MapStateDescriptor<Integer, Integer> mapStateDescriptor = new MapStateDescriptor<>("state", Integer.class, Integer.class); state = getRuntimeContext().getMapState(mapStateDescriptor); }
@Override public void processElement(WaterSensor value, KeyedProcessFunction<String, WaterSensor, String>.Context ctx, Collector<String> out) throws Exception { if (state.get(value.getVc())!=null){ Integer nums = state.get(value.getVc()); state.put(value.getVc(),nums+1); }else { state.put(value.getVc(),1); } out.collect(ctx.getCurrentKey()+":"+state.entries().toString());
} }).print();
env.execute(); } }
|
测试截图:
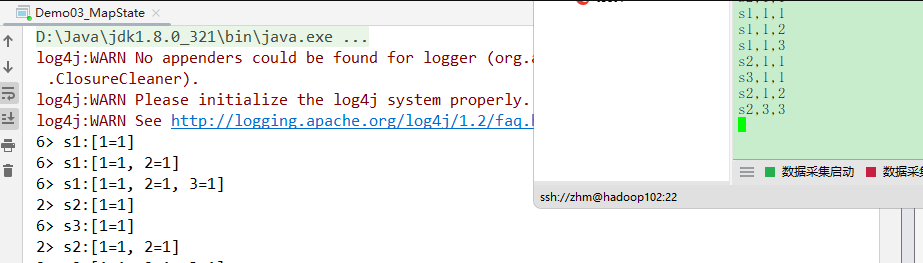
1.2.4、归约状态(ReducingState)
类似于值状态(Value),不过需要对添加进来的所有数据进行归约,将归约聚合之后的值作为状态保存下来。ReducingState 这个接口调用的方法类似于 ListState,只不过它保存的只是一个聚合值,所以调用 .add()
方法时,不是在状态列表里添加元素,而是直接把新数据和之前的状态进行归约,并用得到的结果更新状态。
归约逻辑的定义,是在归约状态描述器(ReducingStateDescriptor)中,通过传入一个归约函数(ReduceFunction)来实现的。这里的归约函数,就是我们之前介绍 reduce 聚合算子时讲到的 ReduceFunction,所以状态类型跟输入的数据类型是一样的。
1 2
| public ReducingStateDescriptor( String name, ReduceFunction<T> reduceFunction, Class<T> typeClass) {...}
|
这里的描述器有三个参数,其中第二个参数就是定义了归约聚合逻辑的 ReduceFunction,另外两个参数则是状态的名称和类型。
1 2 3 4 5 6 7 8 9 10 11 12 13 14 15 16 17 18 19 20 21 22 23 24 25 26 27 28 29 30 31 32 33 34 35 36 37 38 39 40 41 42 43 44 45 46 47 48 49
|
public class Demo04_ReduceState { public static void main(String[] args) throws Exception { Configuration configuration = new Configuration(); configuration.setInteger("rest.port",3333); StreamExecutionEnvironment env = StreamExecutionEnvironment.getExecutionEnvironment(configuration);
env.socketTextStream("hadoop102",9999) .map(new WaterSensorFunction()) .keyBy(WaterSensor::getId) .process(new KeyedProcessFunction<String, WaterSensor, String>() {
private ReducingState<Integer> state;
@Override public void open(Configuration parameters) throws Exception { ReducingStateDescriptor stateDescriptor = new ReducingStateDescriptor<>("state", new ReduceFunction<Integer>() { @Override public Integer reduce(Integer value1, Integer value2) throws Exception { return value1+value2; } }, Integer.class); state = getRuntimeContext().getReducingState(stateDescriptor); }
@Override public void processElement(WaterSensor value, KeyedProcessFunction<String, WaterSensor, String>.Context ctx, Collector<String> out) throws Exception { state.add(value.getVc()); out.collect(ctx.getCurrentKey()+":"+state.get()); } }).print();
env.execute(); } }
|
测试截图:
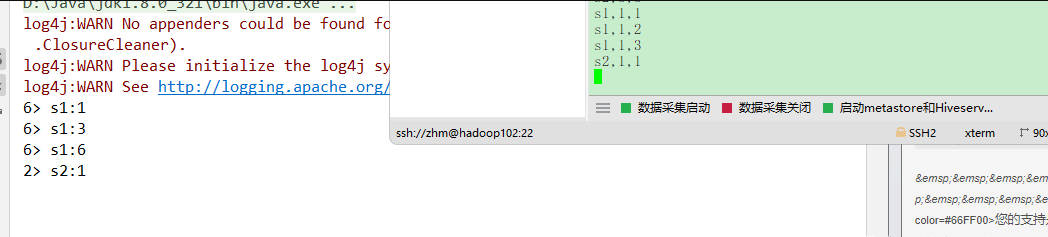
1.2.5、聚合状态(AggregatingState)
与归约状态非常类似,聚合状态也是一个值,用来保存添加进来的所有数据的聚合结果。与 ReducingState 不同的是,它的聚合逻辑是由在描述器中传入一个更加一般化的聚合函数(AggregateFunction)来定义的;这也就是之前我们讲过的 AggregateFunction,里面通过一个累加器(Accumulator)来表示状态,所以聚合的状态类型可以跟添加进来的数据类型完全不同,使用更加灵活。
同样地,AggregatingState 接口调用方法也与 ReducingState 相同,调用 .add()
方法添加元素时,会直接使用指定的 AggregateFunction 进行聚合并更新状态。
1 2 3 4 5 6 7 8 9 10 11 12 13 14 15 16 17 18 19 20 21 22 23 24 25 26 27 28 29 30 31 32 33 34 35 36 37 38 39 40 41 42 43 44 45 46 47 48 49 50 51 52 53 54 55 56 57 58 59 60 61 62 63 64 65 66 67 68 69 70 71 72
|
public class Demo06_AggregatingState { public static void main(String[] args) throws Exception { Configuration configuration = new Configuration(); configuration.setInteger("rest.port",3333); StreamExecutionEnvironment env = StreamExecutionEnvironment.getExecutionEnvironment(configuration);
env.socketTextStream("hadoop102",9999) .map(new WaterSensorFunction()) .keyBy(WaterSensor::getId) .process(new KeyedProcessFunction<String, WaterSensor, String>() {
private AggregatingState<Integer, Double> state;
@Override public void open(Configuration parameters) throws Exception { state = getRuntimeContext() .getAggregatingState( new AggregatingStateDescriptor<>( "state", new AggregateFunction<Integer, Tuple2<Integer, Double>, Double>() { @Override public Tuple2<Integer, Double> createAccumulator() { return Tuple2.of(0, 0d); }
@Override public Tuple2<Integer, Double> add(Integer value, Tuple2<Integer, Double> accumulator) { accumulator.f0 += 1; accumulator.f1 += value; return accumulator; }
@Override public Double getResult(Tuple2<Integer, Double> accumulator) { return accumulator.f1 / accumulator.f0; }
@Override public Tuple2<Integer, Double> merge(Tuple2<Integer, Double> a, Tuple2<Integer, Double> b) { return null; } }, Types.TUPLE(Types.INT, Types.DOUBLE) ) ); }
@Override public void processElement(WaterSensor value, KeyedProcessFunction<String, WaterSensor, String>.Context ctx, Collector<String> out) throws Exception { state.add(value.getVc()); out.collect(ctx.getCurrentKey() +" avgVc:" +state.get()); } }).print();
env.execute(); } }
|
测试截图:
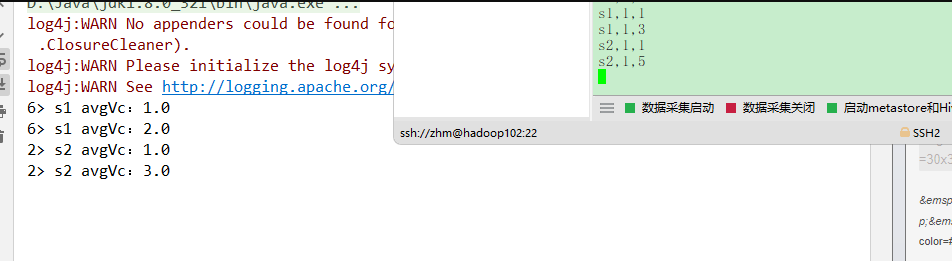
1.2.6、状态生存时间(TTL)
在实际应用中,很多状态会随着时间的推移逐渐增长,如果不加以限制,最终就会导致存储空间的耗尽。一个优化的思路是直接在代码中调用 .clear()
方法去清除状态,但是有时候我们的逻辑要求不能直接清除。这时就需要配置一个状态的“生存时间”(time-to-live,TTL),当状态在内存中存在的时间超出这个值时,就将它清除。
具体实现上,如果用一个进程不停地扫描所有状态看是否过期,显然会占用大量资源做无用功。状态的失效其实不需要立即删除,所以我们可以给状态附加一个属性,也就是状态的“失效时间”。状态创建的时候,设置 失效时间 = 当前时间 + TTL
;之后如果有对状态的访问和修改,我们可以再对失效时间进行更新;当设置的清除条件被触发时(比如,状态被访问的时候,或者每隔一段时间扫描一次失效状态),就可以判断状态是否失效、从而进行清除了。
配置状态的 TTL 时,需要创建一个 StateTtlConfig 配置对象,然后调用状态描述器的 .enableTimeToLive()
方法启动 TTL 功能。
1 2 3 4 5 6 7 8 9
| StateTtlConfig ttlConfig = StateTtlConfig .newBuilder(Time.seconds(10)) .setUpdateType(StateTtlConfig.UpdateType.OnCreateAndWrite) .setStateVisibility(StateTtlConfig.StateVisibility.NeverReturnExpired) .build();
ValueStateDescriptor<String> stateDescriptor = new ValueStateDescriptor<>("my state", String.class);
stateDescriptor.enableTimeToLive(ttlConfig);
|
这里用到了几个配置项:
.newBuilder()
状态 TTL 配置的构造器方法,必须调用,返回一个 Builder 之后再调用 .build()
方法就可以得到 StateTtlConfig 了。方法需要传入一个 Time 作为参数,这就是设定的状态生存时间。
.setUpdateType()
设置更新类型。更新类型指定了什么时候更新状态失效时间,这里的 OnCreateAndWrite 表示只有创建状态和更改状态(写操作)时更新失效时间。另一种类型 OnReadAndWrite 则表示无论读写操作都会更新失效时间,也就是只要对状态进行了访问,就表明它是活跃的,从而延长生存时间。这个配置默认为 OnCreateAndWrite。
.setStateVisibility()
设置状态的可见性。所谓的“状态可见性”,是指因为清除操作并不是实时的,所以当状态过期之后还有可能继续存在,这时如果对它进行访问,能否正常读取到就是一个问题了。这里设置的 NeverReturnExpired 是默认行为,表示从不返回过期值,也就是只要过期就认为它已经被清除了,应用不能继续读取;这在处理会话或者隐私数据时比较重要。对应的另一种配置是 ReturnExpireDefNotCleanedUp,就是如果过期状态还存在,就返回它的值。
除此之外,TTL 配置还可以设置在保存检查点(checkpoint)时触发清除操作,或者配置增量的清理(incremental cleanup),还可以针对RocksDB 状态后端使用压缩过滤器(compaction filter)进行后台清理。这里需要注意,目前的 TTL 设置只支持处理时间。
1 2 3 4 5 6 7 8 9 10 11 12 13 14 15 16 17 18 19 20 21 22 23 24 25 26 27 28 29 30 31 32 33 34 35 36 37 38 39 40 41 42 43 44 45 46 47 48 49 50 51 52 53 54 55 56 57 58 59 60 61 62 63 64 65 66 67 68 69 70 71 72 73 74 75 76 77 78 79 80 81
|
public class Demo09_Ttl{ public static void main(String[] args) throws Exception { Configuration configuration = new Configuration(); configuration.setInteger("rest.port",3333); StreamExecutionEnvironment env = StreamExecutionEnvironment.getExecutionEnvironment(configuration);
env.setParallelism(1);
StateTtlConfig ttlConfig = StateTtlConfig .newBuilder(Time.seconds(15)) .neverReturnExpired()
.setUpdateType(StateTtlConfig.UpdateType.OnReadAndWrite) .build();
env.socketTextStream("hadoop102",9999) .map(new WaterSensorFunction()) .keyBy(WaterSensor::getId) .process(new KeyedProcessFunction<String, WaterSensor, String>() {
private ListState<Integer> listState;
@Override public void open(Configuration parameters) throws Exception { ListStateDescriptor<Integer> listStateDescriptor = new ListStateDescriptor<>("state", Integer.class); listStateDescriptor.enableTimeToLive(ttlConfig);
listState = getRuntimeContext().getListState(listStateDescriptor); }
@Override public void processElement(WaterSensor value, KeyedProcessFunction<String, WaterSensor, String>.Context ctx, Collector<String> out) throws Exception { listState.add(value.getVc()); Iterable<Integer> integers = listState.get(); List<Integer> top3 = StreamSupport.stream(integers.spliterator(), true) .sorted(Comparator.reverseOrder()) .limit(3) .collect(Collectors.toList()); out.collect(ctx.getCurrentKey()+"最新Top3:"+top3); listState.update(top3);
} }).print();
env.execute(); } }
|
测试截图:
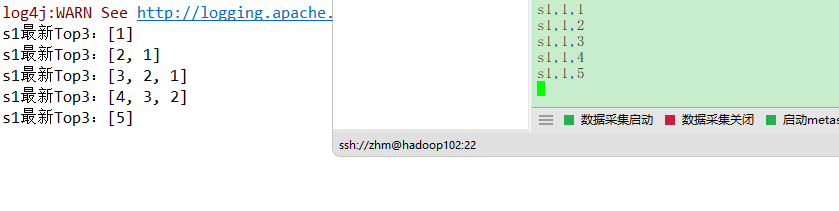
注意:最后一条记录要在上一条记录发送之后15秒之后再发
1.3、算子状态(Operator State)
算子状态(Operator State)就是一个算子并行实例上定义的状态,作用范围被限定为当前算子任务。算子状态跟数据的 key 无关,所以不同 key 的数据只要被分发到同一个并行子任务,就会访问到同一个 Operator State。
算子状态的实际应用场景不如 Keyed State 多,一般用在 Source 或 Sink 等与外部系统连接的算子上,或者完全没有 key 定义的场景。比如 Flink 的 Kafka 连接器中,就用到了算子状态。
当算子的并行度发生变化时,算子状态也支持在并行的算子任务实例之间做重组分配。根据状态的类型不同,重组分配的方案也会不同。
算子状态也支持不同的结构类型,主要有三种:ListState、UnionListState 和 BroadcastState。
1.3.1、列表状态(ListState)
与 Keyed State 中的 ListState 一样,将状态表示为一组数据的列表。
与 Keyed State 中的列表状态的区别是:在算子状态的上下文中,不会按键(key)分别处理状态,所以每一个并行子任务上只会保留一个“列表”(list),也就是当前并行子任务上所有状态项的集合。列表中的状态项就是可以重新分配的最细粒度,彼此之间完全独立。
当算子并行度进行缩放调整时,算子的列表状态中的所有元素项会被统一收集起来,相当于把多个分区的列表合并成了一个“大列表”,然后再均匀地分配给所有并行任务。这种“均匀分配”的具体方法就是“轮询”(round-robin),与之前介绍的 rebanlance 数据传输方式类似,是通过逐一“发牌”的方式将状态项平均分配的。这种方式也叫作“平均分割重组”(even-split redistribution)。
算子状态中不会存在“键组”(key group)这样的结构,所以为了方便重组分配,就把它直接定义成了“列表”(list)。这也就解释了,为什么算子状态中没有最简单的值状态(ValueState)。
案例实操:在 map 算子中计算数据的个数。
1 2 3 4 5 6 7 8 9 10 11 12 13 14 15 16 17 18 19 20 21 22 23 24 25 26 27 28 29 30 31 32 33 34 35 36 37 38 39 40 41 42 43 44 45 46 47 48 49 50 51 52 53 54 55 56 57 58 59 60 61 62 63 64 65
| public class OperatorListStateDemo { public static void main(String[] args) throws Exception { StreamExecutionEnvironment env = StreamExecutionEnvironment.getExecutionEnvironment(); env.setParallelism(2);
env .socketTextStream("hadoop102", 7777) .map(new MyCountMapFunction()) .print();
env.execute(); }
public static class MyCountMapFunction implements MapFunction<String, Long>, CheckpointedFunction {
private Long count = 0L; private ListState<Long> state;
@Override public Long map(String value) throws Exception { return ++count; }
@Override public void snapshotState(FunctionSnapshotContext context) throws Exception { System.out.println("snapshotState..."); state.clear(); state.add(count); }
@Override public void initializeState(FunctionInitializationContext context) throws Exception { System.out.println("initializeState..."); state = context .getOperatorStateStore() .getListState(new ListStateDescriptor<Long>("state", Types.LONG));
if (context.isRestored()) { for (Long c : state.get()) { count += c; } } } } }
|
1.3.2、联合列表状态(UnionListState)
与 ListState 类似,联合列表状态也会将状态表示为一个列表。它与常规列表状态的区别在于,算子并行度进行缩放调整时对于状态的分配方式不同。
UnionListState 的重点就在于“联合”(union)。在并行度调整时,常规列表状态是轮询分配状态项,而联合列表状态的算子则会直接广播状态的完整列表。这样,并行度缩放之后的并行子任务就获取到了联合后完整的“大列表”,可以自行选择要使用的状态项和要丢弃的状态项。这种分配也叫作“联合重组”(union redistribution)。如果列表中状态项数量太多,为资源和效率考虑一般不建议使用联合重组的方式。
使用方式同 ListState,区别在如下部分:
1 2 3
| state = context .getOperatorStateStore() .getUnionListState(new ListStateDescriptor<Long>("union-state", Types.LONG));
|
1.3.3、广播状态(BroadCastState)
有时我们希望算子并行子任务都保持同一份“全局”状态,用来做统一的配置和规则设定。这时所有分区的所有数据都会访问到同一个状态,状态就像被“广播”到所有分区一样,这种特殊的算子状态,就叫作广播状态(BroadcastState)。
因为广播状态在每个并行子任务上的实例都一样,所以在并行度调整的时候就比较简单,只要复制一份到新的并行任务就可以实现扩展;而对于并行度缩小的情况,可以将多余的并行子任务连同状态直接砍掉——因为状态都是复制出来的,并不会丢失。
1 2 3 4 5 6 7 8 9 10 11 12 13 14 15 16 17 18 19 20 21 22 23 24 25 26 27 28 29 30 31 32 33 34 35 36 37 38 39 40 41 42 43 44 45 46 47 48 49 50 51 52 53 54 55 56 57 58 59 60 61 62 63 64 65 66 67 68 69 70 71 72 73
|
public class Demo04_BroadCastState { public static void main(String[] args) throws Exception { Configuration configuration = new Configuration(); configuration.setInteger("rest.port",3333); StreamExecutionEnvironment env = StreamExecutionEnvironment.getExecutionEnvironment(configuration);
env.setParallelism(2);
env.enableCheckpointing(2000);
SingleOutputStreamOperator<WaterSensor> dataDS = env .socketTextStream("hadoop102", 9999) .map(new WaterSensorFunction());
SingleOutputStreamOperator<MyConf> configDS = env.socketTextStream("hadoop102", 9998) .map(new MapFunction<String, MyConf>() { @Override public MyConf map(String value) throws Exception { String[] split = value.split(","); return new MyConf(split[0], split[1]); } });
MapStateDescriptor<String, MyConf> mapStateDescriptor = new MapStateDescriptor<>("config", String.class, MyConf.class);
BroadcastStream<MyConf> confBroadcastStream = configDS.broadcast(mapStateDescriptor);
dataDS.connect(confBroadcastStream) .process(new BroadcastProcessFunction<WaterSensor, MyConf, WaterSensor>() { @Override public void processElement(WaterSensor value, BroadcastProcessFunction<WaterSensor, MyConf, WaterSensor>.ReadOnlyContext ctx, Collector<WaterSensor> out) throws Exception { ReadOnlyBroadcastState<String, MyConf> broadcastState = ctx.getBroadcastState(mapStateDescriptor); MyConf myConf = broadcastState.get(value.getId());
value.setId(myConf.getName()); out.collect(value);
} @Override public void processBroadcastElement(MyConf value, BroadcastProcessFunction<WaterSensor, MyConf, WaterSensor>.Context ctx, Collector<WaterSensor> out) throws Exception { BroadcastState<String, MyConf> broadcastState = ctx.getBroadcastState(mapStateDescriptor); broadcastState.put(value.id,value); } }).print(); env.execute(); }
@Data @AllArgsConstructor @NoArgsConstructor public static class MyConf{ private String id; private String name; } }
|
测试截图:
